Opportunities for Innovation in AI in Education
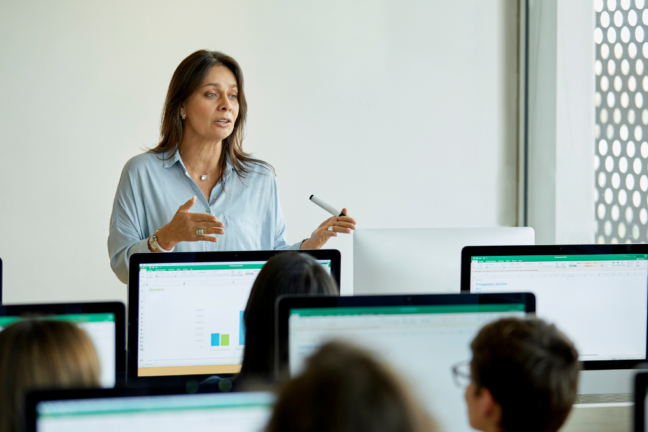
This article was adapted from a presentation given at the 2021 Next Generation by Design (NGBD) Symposia Series, sponsored by DXtera Institute and Riiid Labs.
It all begins with this question: “Why spur innovation in artificial intelligence (AI) in education?”
We want to run a little faster toward a future in which we can address inequities by advancing the accessibility, availability, and affordability of education. AI in education is often seen as an opportunity to do just this. Yet, development and adoption of AI in education seems to lag behind other industries. What is it that would spur the horse into action? What would speed it along in its journey to that future?
By aligning different elements in the educational technology market, edtech creators can reduce friction.
Do they include incompatible technology, school data systems, functionality of programs, etc.? Is the data format disparate? Or is the type of data collected disparate? While the challenge of reducing friction from disparate components in the educational ecosystem is highly complex, one singular area of focus that could advance this horse to a trot may be to open it up. Make available open data for training and validation, use open-source software for methods and algorithms, establish and build to interoperability standards, and increase transparency on how to evaluate AI tools and their impact on learning. Indeed, some of the work of the Adaptive Instructional Systems (AIS) Consortium DXtera Institute, the Riiid Labs Artificial Intelligence Education (AIEd) Challenge, and others is focused on exactly this.
Even with the best of intentions, our efforts might fail.
A possible failure would not be because we can’t technically figure it out but because we can’t spur innovation on AI tools that don’t solve important problems, we don’t represent the people using those tools, or worse, we make decisions that have negative consequences for individuals and communities. The hardest work can be the most rewarding. And, in this case, our challenges are our greatest opportunities.
As we do this work, here are five opportunities for humans to address.
What data are missing?
We are all aware of the scenarios in which incomplete training sets rendered an AI-enabled product useless for some users. But we have to dig a little deeper than simply thinking about who may not be represented in a specific dataset. We have to ask questions such as:
- What data haven’t been collected?
- What data will not be collected?
- Who decided which data to collect in the first place?
- What problems were they trying to solve?
These can be important reflections of power structures in our societies. Alone, data sets are not neutral, and one could further make the claim that they are not inherently objective because they are the product of these decisions. The book Data Feminism by Catherine D’Ignazio and Lauren F. Klein provides some excellent examples of this.
What will we decide to prioritize as we build?
- Synthetic and simulated datasets
- Semantic information
- Contextual data
What will be deprioritized? Who will those decisions impact?
What and for whom do we optimize?
AI is in the business of optimization. Recent studies examining teachers’ use of adaptive instructional systems in classrooms have found that the systems are often relegated to supplementary instruction, with usage far lower than the providers of those solutions advise to see positive effects. Time is seen as a serious constraint to teachers’ use of these systems, along with other challenges, such as connected versus disconnected interventions, a topic often discussed in intervention literature.
Indeed, coherence among what is happening in the classroom, in our instructional technologies, and in the systems which establish what it means to be successful is going to be paramount. How do we achieve that? We can start by getting explicit about what a given AI solution is meant to optimize: Mastery versus opportunity to learn? A test score at a given point in time? The speed with which a learner grows?
Edmentum’s Learning Design and Research and Learning Engineering teams have been working closely on how we approach optimizing adaptive instructional systems within the core learning day and solid pedagogic frameworks. This paper, presented at the International Conference on Human-Computer Interaction, proposes a conceptual model for hybrid adaptive instructional and assessment systems (AIAS).
What will we accept as evidence of efficacy?
What Works Clearinghouse (WWC, a highly regarded reviewer of educational research, offers explicit guidance on how the effectiveness of an educational intervention can be established. The gold standard is a randomized control trial that takes a baseline measure and compares differences in control to intervention samples on the same measure following intervention. The simplest versions of these studies cost around $200,000. I’d suggest that one of the components to consider in terms of removing barriers to AI in education is to consider infrastructure that could streamline these types of studies.
It isn’t easy. As evidenced in conversations at the National Council on Measurement in Education’s (NCME’s) 2021 annual meeting, we must first know which outcomes matter before we can determine how well we can and do measure those outcomes.
Then, we ask the other pertinent questions: How sensitive are those measures to the interventions we want to test? How do we weigh the consequences of false positives? Of false negatives? How do we, alongside our open code, represent the implementations in which a solution has worked? Or has not worked? Are we brave enough to point to the things that didn’t work? Or to share when we don’t know if a particular solution will work in a particular context?
In AI, we often strive for incrementally better predictive accuracy results. Please, let’s not stop there. Let’s work to finish the story about what works and for whom and under what conditions.
Who gets to decide?
To borrow a phrase popularized by Lin-Manuel Miranda’s Hamilton, who gets to be in “the room where it happens?” Who will be deciding on the components? Who decides what it means to align them? Who decides what we strive for in optimization., And how accurate is accurate enough?
Consider this simple example for the impact our deciders could have. According to the U.S. Bureau of Labor Statistics, today women earn only 18 percent of computer science bachelor’s degrees in the United States, and the ratio of women to men working in computer-science-related professions has declined since the 1990s to about one in five. How would the implementations for AI in education shift if more women contributed to building these solutions?
This past March, the Institute for Ethical AI in Education released the creatively named report, “The Ethical Framework for AI in Education.” Some of the items in this framework point to being able to explicate decision-making in the development of the AI resource, in addition to the impact of using it.
It’s hard. At Edmentum, we work to hire a diverse team with many perspectives, and bring perspectives of others into our work. We have to keep working toward bringing more people, perspectives, and priorities into “the room where it happens.”
How will humans play?
At Edmentum, we live by the mantra #EducatorFirst. This means that we consider and involve the educator in all the work we do. As we build AI solutions in education, we examine how we purposefully build humans into the loop.
What are your assumptions about the role and agency of the teacher, the role and agency of the student, and the roles and agency of other actors within the educational ecosystem? How can we embed human-centered design into our practices, and how can we ensure that we are using every opportunity to do it? How do we test individual components with humans in the loop?
That concludes our list of five—call them considerations, difficulties, or challenges. We prefer to call them opportunities, as we work to open up and align resources, remove friction, and spur innovation.
There is a saying that you can lead a horse to water, but you can’t make it drink.
Fastness isn’t everything. No matter how quickly we get the horse there by digging in our spurs, if the water doesn’t taste good, the horse won’t drink.
We can spur innovation on AI tools that don’t solve the important problems, that don’t represent the people using those tools, and worse, that make automated decisions that have disastrously negative consequences for individuals and communities. Or, we can purposefully and inclusively address these five opportunities. In addition to accelerating development with a spur in the side, let’s be certain to make the water taste good.